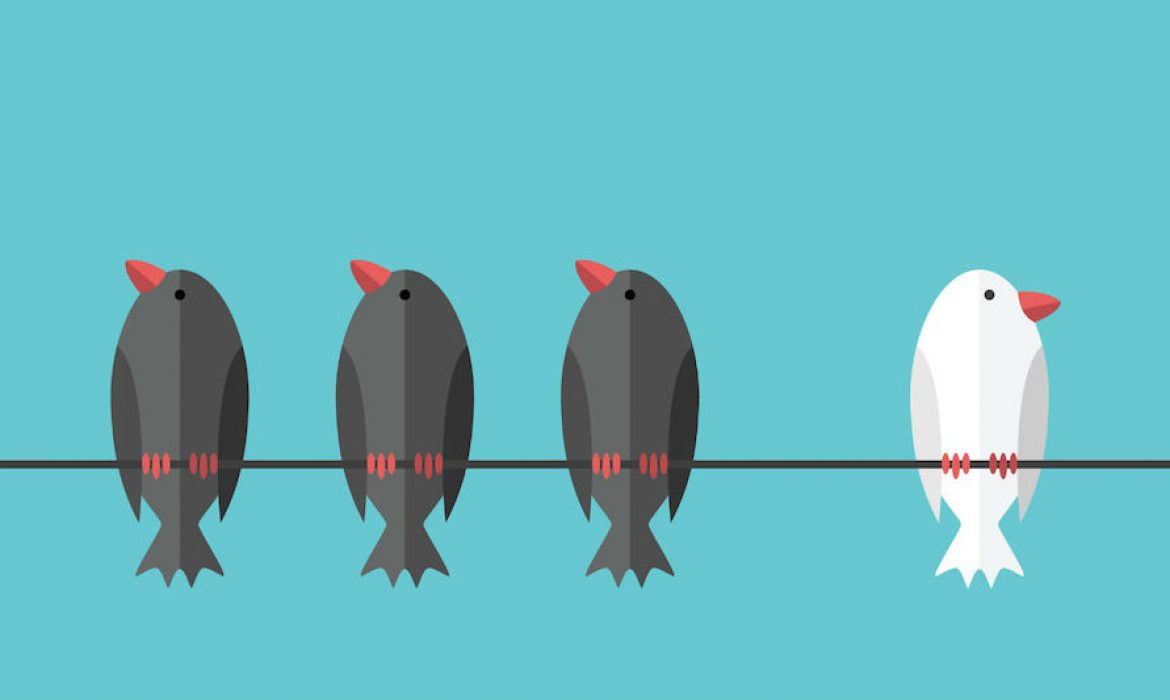
Algorithm design, data and implementation are key to minimizing prejudice.
Algorithms, especially those that utilize deep learning in some manner, are notorious for being opaque. To be clear, this means that when you ask a deep learning algorithm to answer a question, the algorithm gives you an answer without any explanation of how it came to that conclusion. It does not show its work; you simply ask it a question and it generates an answer, like a mysterious oracle.
As author Scott Fulton III points out, “We’ve created systems that draw mostly, though never entirely, correct inferences from ordinary data, by way of logic that is by no means obvious.” Should these systems be trained on faulty or incomplete data, they have the capacity to further entrench existing biases and perpetuate discriminatory behavior.
Bias isn’t inherent
I believe that there are ways that we can use deep learning to help eliminate these inequalities, but it requires organizations to interrogate their existing practices and data more deeply. We are beginning to see the biased and hurtful results of this in the advertising industry, as deep learning is increasingly used to decide what ads you see. Everyone needs to exhibit greater awareness about how their ads are perceived, as well as who is viewing them.
The problem that many marketers currently face is that they rely on third-party platforms to determine who their ad is shown to. For example, while advertising platforms like Facebook allow marketers to roughly sketch out their target audience, it is ultimately up to the algorithm itself to identify the exact users who will see the ad. To put it another way, a company might put out an ad that is not targeted to a specific age group, ethnicity, or gender, and still find that certain groups are more likely to see their ads than others.
How algorithms can perpetuate bias
Earlier this year, a team from Northeastern University decided to carry out a series of experiments designed to identify the extent to which Facebook’s ad delivery is skewed along demographic lines. While Facebook’s algorithm does allow advertisers to target certain demographics, age groups, and genders with precision, the researchers wanted to see whether giving the algorithm neutral targeting parameters for a series of ads would also result in a similar skew, despite not targeting any single specific group; in other words, whether people with particular demographic characteristics were more likely to see certain ads than others.
To test this hypothesis, the researchers ran a series of ads that were targeted to the exact same audience and had the same budget, but used different images, headlines, and copy. They found that ads with creative stereotypically associated with a specific group (ie. bodybuilding for men or cosmetics for female audiences) would overwhelmingly perform better amongst those groups, despite not being set up to target those audiences specifically.
Researchers also discovered that, of all the creative elements, the image was by far the most important in determining the ad’s audience, noting that “an ad whose headline and text would stereotypically be of the most interest to men with the image that would stereotypically be of the most interest to women delivers primarily to women at the same rate as when all three ad creative components are stereotypically of the most interest to women.”
These stereotypes become much more harmful when advertising housing or job openings, to name a few sensitive areas. As the researchers from Northeastern discovered, job postings for secretaries and pre-school teachers were more likely to be shown to women, whereas listings for taxi drivers and janitors were shown to a higher percentage of minorities.
Why does this happen? Because Facebook’s algorithm optimizes ads based on a market objective (maximizing engagement, generating sales, garnering more views, etc.), and does not pay as much attention to minimizing bias. As a result, Karen Hao notes in the MIT Technology Review, “if the algorithm discovered that it could earn more engagement by showing more white users homes for purchase, it would end up discriminating against black users.”
There are other approaches
However, the algorithm does this because it has been taught to approach the issue from a purely economic perspective. If, on the other hand, it had been trained from the start to be aware of potential discrimination, and to guard against it, the algorithm could end up being much less biased than if marketers were left to their own devices. Brookings Institute suggests that developers of algorithms create what they term a “bias impact statement” beforehand, which is defined as “a template of questions that can be flexibly applied to guide them through the design, implementation, and monitoring phases,” and whose purpose is to “help probe and avert any potential biases that are baked into or are resultant from the algorithmic decision.”
Take, for instance, mortgage lending. Research has shown that minorities, especially African Americans and Latinos, are more likely to be denied mortgages even when taking into account income, the size of the loan, and other factors. An algorithm that relies solely on data from previous loans to determine who to give a mortgage to would only perpetuate those biases; however, one that is designed specifically to take those factors into account could end up creating a much fairer and more equitable system.
While there is clearly more work to be done to make sure that algorithms do not perpetuate existing biases (or create new ones), there is ample research out there to suggest a way forward — namely, by making marketers and developers aware of the prejudices inherent in the industry and having them take steps to mitigate those preferences throughout the design, data cleansing, and implementation process. A good algorithm is like wine; as it ages, it takes on nuance and depth, two qualities that the marketing industry sorely needs.
___
source: Marketing Land